Top 10 challenges of big data
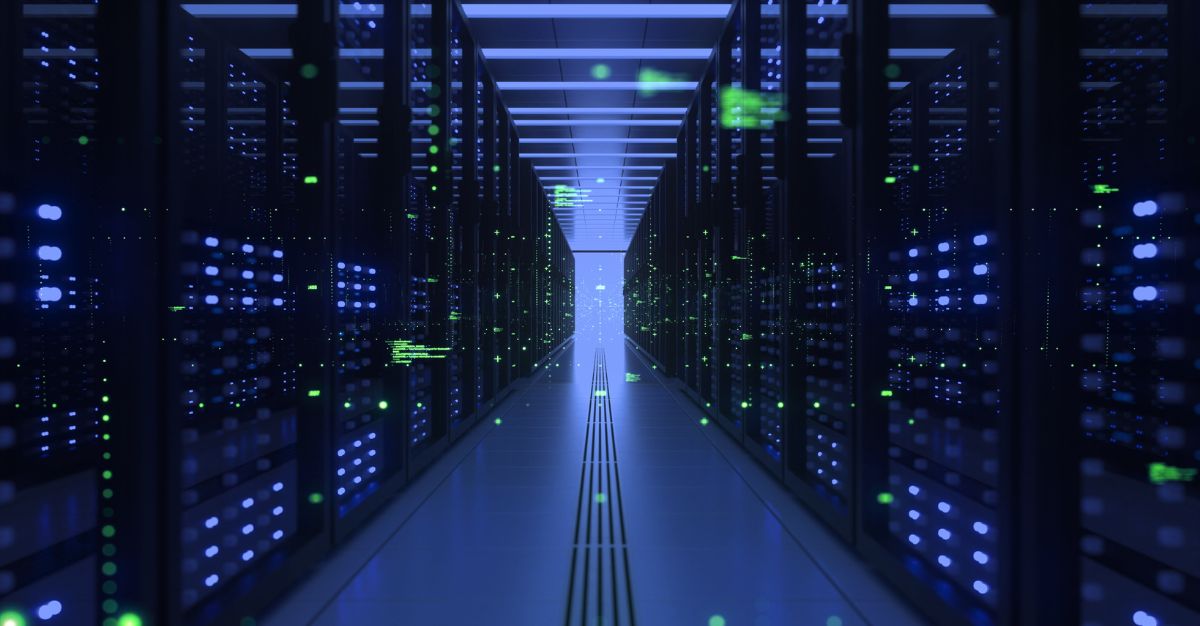
Working with big data can be a steep learning curve–and it’s easy to get it wrong. Knowing the challenges will help you avoid common pitfalls that could seriously affect your business.
- Data quality issues
Data quality is crucial to any big data project. To make sure your system is collecting accurate data and getting rid of any that’s out of date, you need to be able to spot and fix data quality issues at every stage of the data lifecycle.
Collection: Make sure you’re collecting data from the right sources at the right time. Storage: Make sure it’s stored in the right place and accessible. Maintenance: Make sure the data is validated and moved to the right location so the right teams can work on it when they need to. Data usage: Time to make decisions based on your data. Double-check there are no errors in the previous three steps leaving you with faulty data. Data cleaning: Delete data that’s no longer valid or useful, and archive data that is.- Long system response times
Your system should be able to process new data quickly–delays can be costly when a report is due. Look at how your data is organised, and try to store the most important data close to the surface where you can grab it quickly. Or upgrade your system if your current one has reached its limit of scalability.
- Data integration
Data integration is complex and easy to get wrong. You have to integrate data to be able to use it. Big data platforms can help you store large quantities of data, but you need to ensure that it’s easy to access. For example, if you’re using the cloud to store all your data, make sure it’s always accessible in one central location.
- Scaling your system
Big data systems are usually easy to scale–but if you don’t go in with a clear plan from the start, you’ll soon end up with a confusing mess of data. Decide what types of data you’ll collect, how you’ll store and use them, and how you’ll cycle out old data. Organising data using Parquet files tends to be more cost-effective than CSV dumps. Speaking of which…
- When your data bank is breaking the bank
Cloud-based solutions make it so easy to save data that you may quickly find yourself needing way more storage than you budgeted for. Avoid this by implementing fine controls on queries so they only save the most necessary data.
- Data governance issues
Another thing you need to do at the start of any new process: build in governance rules. Governance issues will become much harder to manage as your data grows, and you may end up accidentally hindering the type of data access you were actually looking for.
- Maintenance costs
It costs money to keep the systems maintaining your data in working order and upgrade them when they become outdated. However, upgrading can actually save you money, particularly if you go for cloud-based platforms, many of which offer pay-as-you go solutions. Or if you find yourself blessed with more bells and whistles than you need, you can save by downgrading to a simpler system.
- Inaccurate analyses
There are two main reasons your data analysis might be giving you inaccurate results:
Poor data quality System defectsWe’ve already covered data quality, but you also need to make sure you test your platform and verify every part of the development to spot any problems and make sure your data is being handled correctly.
- Data silos
Can everyone in your organisation access the data they need? If not, you’ve got a problem with data silos, and it’s slowing everyone down. The most common reason for data silos is storing data on separate databases. Consider a central cloud-based solution instead.
- Unprotected and unsecured data
With cybercrime on the rise, it’s more important than ever to keep your data secure. Make sure whatever platform you use has good security that protects you from infiltrators, viruses, and malware.